AI Summary
Med-Gemini is a family of models fine-tuned for medicine that outperforms GPT-4 in medical benchmarks, achieving 91.1% accuracy on the MedQA (USMLE) benchmark and surpassing other LLMs in multimodal medical benchmarks. It builds upon Gemini models' capabilities in processing information from various modalities and demonstrates strong long-context reasoning and self-training features to enhance clinical reasoning and utilization of external information.
May 06 2024 16:51The field of medicine is a complex and multifaceted endeavor that requires clinicians to possess a wide range of skills and knowledge. From patient consultations to complex case analysis, medical professionals must effectively communicate, reason, and collaborate to provide the best possible care. While artificial intelligence (AI) systems have shown promise in assisting with individual medical tasks, there is still significant room for improvement in terms of reasoning, multimodal understanding, and long-context comprehension.
Enter Med-Gemini, a family of models fine-tuned and specialized for medicine, built upon the highly capable multimodal Gemini models. Instead of attempting to build a single generalist medical AI system, Med-Gemini introduces a family of models, each optimized for different capabilities and application-specific scenarios, taking into account factors such as training data, compute availability, and inference latency.
Outperforming GPT-4 and Doctors
Med-Gemini's performance on medical benchmarks is nothing short of impressive. It established new state-of-the-art results on 10 out of 14 benchmarks, surpassing the GPT-4 model family in every comparable benchmark. On the MedQA (USMLE) benchmark, Med-Gemini achieved a remarkable 91.1% accuracy, outperforming Google's previous medical LLM, Med-PaLM 2, by 4.5%.
Med-Gemini's Multimodal Capabilities
Med-Gemini builds upon the foundational Gemini models, which excel at processing information from various modalities, including text, images, videos, and audio. These models are proficient in language and conversation, understanding diverse information, and engaging in long-context reasoning. Med-Gemini takes these capabilities a step further by fine-tuning them specifically for medical applications.
Med-Gemini attains SoTA performance on 5 out of 7 multimodal medical benchmarks. It demonstrate the effectiveness of multimodal medical fine-tuning and the ability to customize to novel medical modalities such as electrocardiograms (ECGs). Med-Gemini also exhibits strong long-context reasoning capabilities, attaining SoTA on challenging benchmarks such as “needle-in-the-haystack” tasks in lengthy electronic health records or benchmarks for medical video understanding.
Self-Training and Web Search
One of Med-Gemini's standout features is its ability to self-train and leverage web search capabilities to enhance clinical reasoning. The left panel illustrates the self-training with search framework used to fine-tune Med-Gemini-L 1.0 for advanced medical reasoning and use of web search. This framework iteratively generates reasoning responses (CoTs) with and without web search, improving the model’s ability to utilize external information for accurate answers.
The right panel illustrates Med-Gemini-L 1.0’s uncertainty-guided search process at inference time. This iterative process involves generating multiple reasoning paths, filtering based on uncertainty, generating search queries to resolve ambiguity, and incorporating retrieved search results for more accurate responses.
Long-Context Processing and EHR Understanding
Med-Gemini's long-context processing capabilities open up new frontiers in medical AI applications. The model demonstrated its ability to retrieve specific information from lengthy electronic health records (EHRs) in a "needle-in-a-haystack" task. It successfully retrieved relevant mentions of rare and subtle medical conditions, symptoms, or procedures from extensive clinical notes, outperforming the state-of-the-art method in recall.
Here is an example of Med-Gemini-M 1.5’s long-context capabilities on long EHR understanding. It performs a two-step process for determining whether a patient has a history of a specific condition based on their extensive EHR records.
- Retrieval: It identifies all mentions of “hypothermia” within the EHR notes, providing direct quotes and note IDs for each mention.
- Deciding the existence: It then evaluates the relevance of each retrieved mention, categorizing them as explicit confirmation, strong indication, or relevant mention of hypothermia.
Based on this analysis, the model concludes that the patient does have a history of hypothermia, providing clear reasoning for its decision.
Real-World Conversations and Assistance
In tests simulating real-world scenarios, Med-Gemini showcased its diagnostic dialogue capabilities. When presented with an itchy skin lump by a patient user, the model asked appropriate follow-up questions, correctly diagnosed the rare lesion, and provided recommendations. It also demonstrated its ability to interpret chest X-rays for physicians and generate plain English reports for patients, highlighting its potential to assist clinicians and enhance patient communication.
In this interaction, Med-Gemini-M 1.5 asks for a picture when it is not provided, arrives at the right diagnosis efficiently, explains the reasoning by integrating the relevant visual features and other gathered patient symptoms, answers questions about treatment options while deferring aptly to experts for the final decision.
Responsible AI and Future Directions
While Med-Gemini's initial capabilities are promising, the researchers acknowledge that further work is needed. They plan to incorporate responsible AI principles, including privacy and fairness, throughout the model development process. Ensuring the reliability and safety of these systems is paramount as they advance in capabilities.
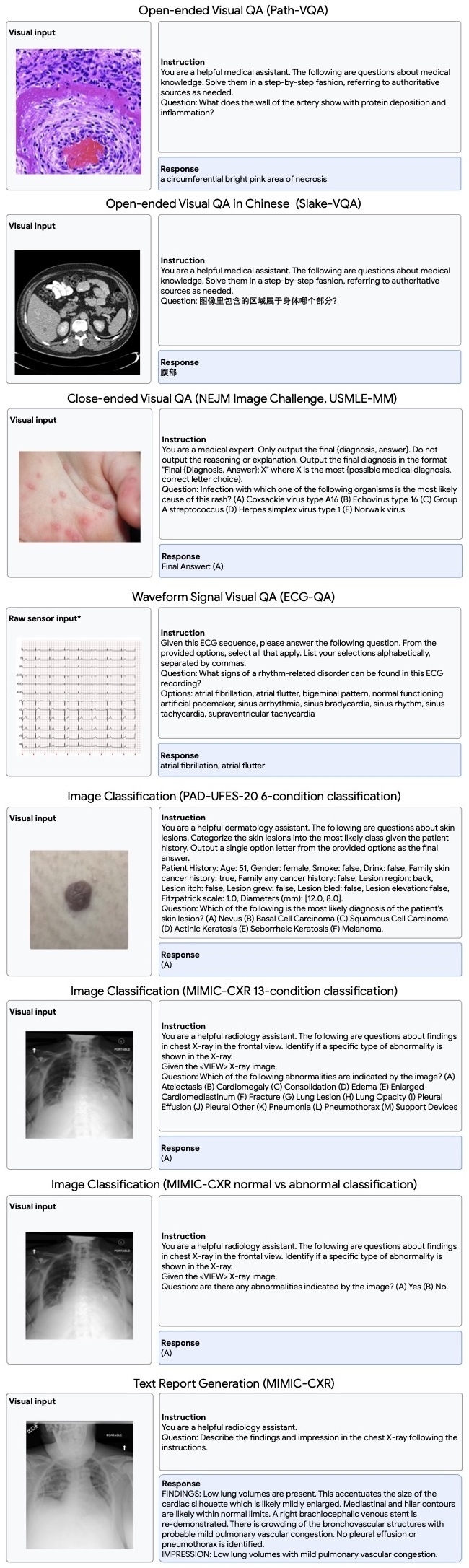
The development of Med-Gemini has the potential to significantly advance the field of medical AI, enabling more intuitive and helpful assistive tools for clinicians and patients alike. By leveraging the multimodal capabilities of the Gemini models and fine-tuning them for specific medical applications, Med-Gemini can help address the challenges of clinical reasoning under uncertainty, effective collaboration with clinicians, and the integration of complex multimodal medical data. As a result, Med-Gemini could lead to improved patient outcomes, enhanced clinical decision-making, and more efficient healthcare delivery, paving the way for a new era of AI-driven medicine.
Full Report:
Capabilities of Gemini Models in MedicineRecent Posts